Artificial intelligence (AI) is rapidly transforming our world, impacting everything from the social media content to the medical diagnoses. While AI holds immense potential, it also requires responsible development.
How do you feel if being denied a loan due to biased AI decisions? Frustrating, right? To ensure AI benefits everyone fairly and safely, we must adhere to core principles. This guide dives deep into the four key principles of Responsible AI: fairness, transparency, accountability, and security & privacy.
>> Read more:
- Will AI Replace Software Engineers Altogether?
- What is the Responsibility of Developers using Generative AI?
- Which Case Would Benefit from Explainable AI Principles?
What is Responsible AI?
Responsible AI refers to the ethical and accountable development and deployment of artificial intelligence systems. It ensures that AI technologies are developed and used in ways that are fair, transparent, accountable, and protective of privacy and security. This approach prioritizes the welfare of individuals and society, preventing harm and promoting trust in AI applications.
Principle 1: Fairness
Fairness in AI is all about ensuring that AI systems make decisions that are just and unbiased. This doesn't mean achieving perfect equality in every outcome, but rather preventing the system from systematically favoring or disadvantaging certain groups based on factors like race, gender, or socioeconomic status.
2 Popular Types of Bias
Bias can enter AI systems in two main ways:
- Data Bias: This occurs when the training data used for the AI model is itself biased.
- Algorithmic Bias: This bias is inherent to the way the AI algorithm is designed.
Examples of Bias
Data Bias:
- Loan Approval System: A bank trains its AI for loan approvals using historical data. If this data shows a higher rate of defaults from borrowers in a particular zip code, the AI might unfairly deny loans to new applicants from that area, regardless of their individual creditworthiness.
- Medical Diagnosis Tool: An AI tool for diagnosing illnesses is trained on medical data primarily from wealthy, urban areas. This can lead to misdiagnosis for patients from rural areas or those with lower incomes, whose health profiles might differ significantly.
- Image Recognition Software: An AI trained on a dataset with mostly images of people with light skin might fail to recognize faces of people with darker skin tones, leading to inaccurate or biased results.
Algorithmic Bias:
- Content Filtering System: An AI filter designed to remove offensive content from social media platforms might be programmed to flag slang terms more commonly used by certain demographics, unfairly censoring their speech.
- News Recommendation Engine: An AI that recommends news articles to users might prioritize sensationalized content over in-depth reporting, regardless of its truthfulness, leading to the spread of misinformation.
- Self-Driving Car Algorithm: A self-driving car's decision-making algorithm might prioritize protecting the car itself over pedestrians or cyclists, potentially leading to biased decision-making in critical situations.
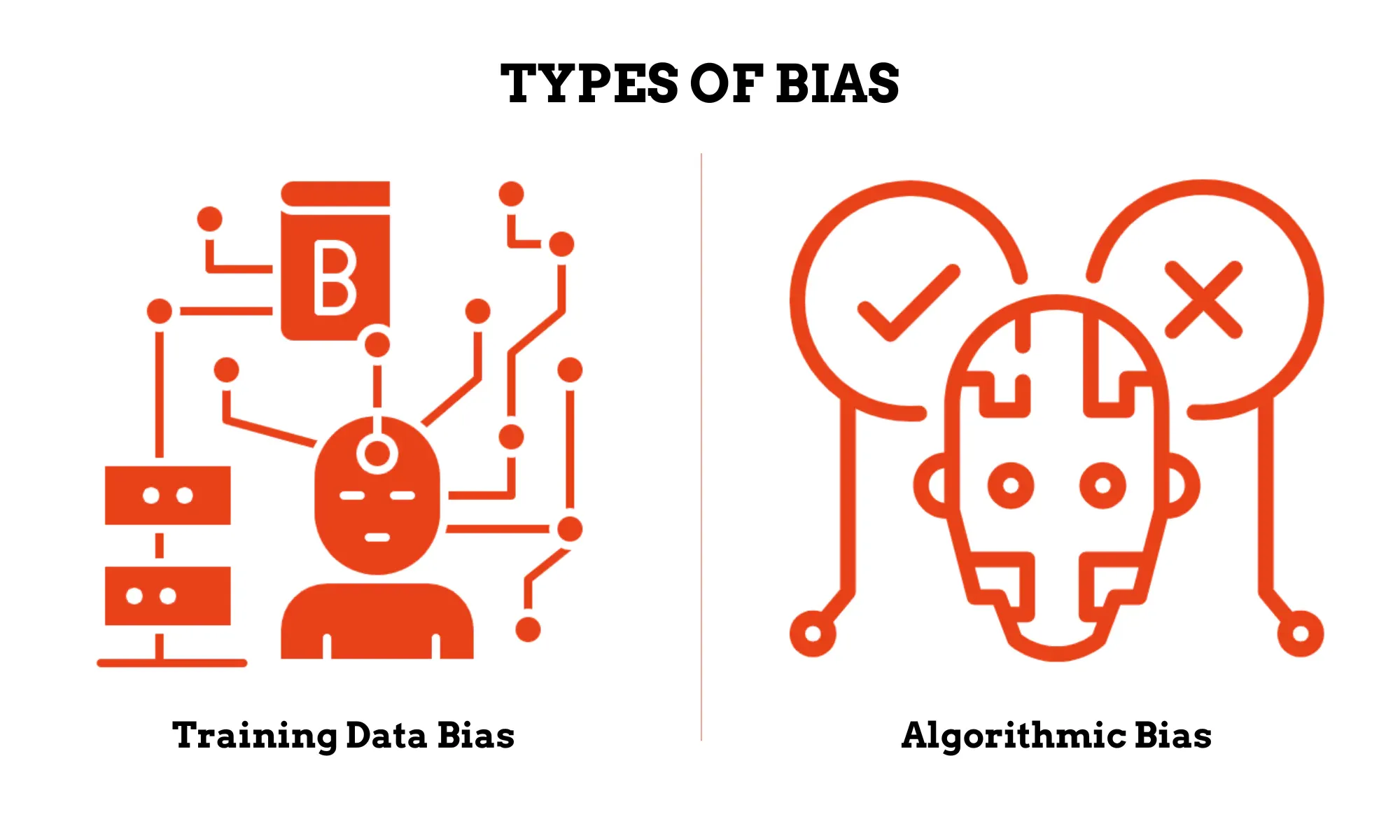
Strategies for Mitigating Bias
Fortunately, there are steps to take towards fairer AI:
- Train AI models on data that have to reflect the real world's diversity.
- Regularly evaluate AI systems for bias through metrics.
- Always maintain human oversight.
- Apply techniques like debiasing algorithms and fairness-aware model design to reduce bias.
Achieving perfect fairness in AI is an ongoing challenge. Researchers are constantly developing new methods to identify and mitigate bias. By understanding the types of bias, their potential harms, and the strategies to combat them, we can ensure AI remains a force for good that benefits everyone equally.
Principle 2: Transparency
Transparency in AI means making the decision-making processes of AI systems clear and understandable. It involves openly sharing how AI models work, what data they use, and how decisions are made, so users can trust and verify the outcomes.
Transparency is crucial for building trust in AI systems. When users understand how AI decisions are made, they are more likely to trust and accept those decisions. Transparency also helps in identifying and correcting errors or biases in AI systems, ensuring fairer outcomes.
Different Levels of Transparency
Explainability: This level focuses on providing users with an understanding of the rationale behind an AI's decision. It aims to answer the question: "Why did the AI make this decision?". Here are some techniques for explainability:
- Explain which factors in the data most influenced the AI's decision.
- Show users how a slight change in the input data could have resulted in a different outcome.
- Provide similar examples where the AI made a similar decision, helping users understand the decision-making patterns.
Interpretability: This goes beyond explaining individual decisions and aims to make the entire AI model more understandable. It focuses on the question: "How does the AI work?". Here's how interpretability can be achieved:
- Simpler Models: Using less complex algorithms can make them easier to understand and interpret. However, simpler models may not achieve the same level of performance as complex ones.
- Visualization Techniques: Tools like decision trees or rule sets can visually represent the decision-making logic of the AI model.
Human Oversight: This level focuses on involving humans in the decision-making process of AI systems. It ensures that even if the AI's internal workings are opaque, there's a human element for accountability and understanding. Here's how human oversight works:
- Humans review and approve the AI's recommendations before they are implemented.
- AI developers can create explanation tools specifically designed for human reviewers, providing them with insights into the AI's decision-making process.
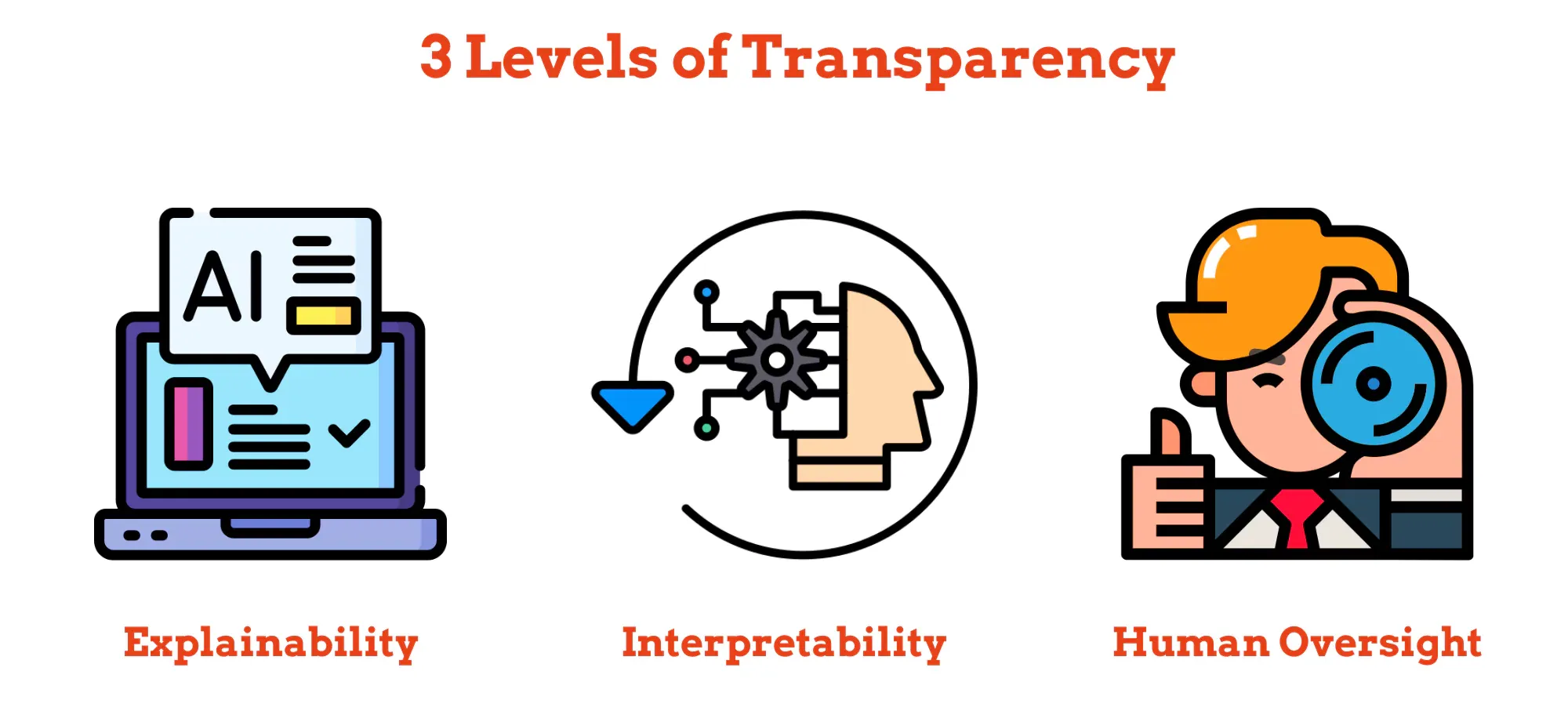
Examples of Transparency
- Loan Approval Systems: Transparency could involve explaining why a loan was approved or denied based on specific criteria (e.g., credit score, income, debt-to-income ratio). This allows applicants to understand the rationale behind the decision and potentially improve their chances for future approval.
- Facial Recognition Software: Transparency can involve informing users about the accuracy of the system and the potential for errors. It could also allow users to opt-out of having their facial data used for recognition purposes.
- Content Moderation AI: Transparency relates to providing users with the specific criteria used to identify inappropriate content. Additionally, users could be informed about the possibility of their content being flagged incorrectly and offered an appeals process.
Strategies for Achieving Transparency
- Developing AI models that can be easily interpreted by humans. Techniques such as decision trees or rule-based models are often more transparent than complex neural networks.
- Conducting regular audits of AI systems to ensure they are functioning as intended and to identify any hidden biases or errors.
- Creating user interfaces that provide clear and simple explanations of AI decisions, making it easier for users to understand and trust the system.
Researchers are constantly developing new techniques for explainable AI (XAI). As XAI tools become more sophisticated, transparency in AI will continue to improve. By prioritizing transparency, we can build AI systems that are not only powerful but also trustworthy and accountable to the people they serve.
Principle 3: Accountability
Accountability in AI ensures that developers and organizations are responsible for the behavior and impacts of their AI systems. This principle aims to establish clear roles and responsibilities for managing AI systems and addressing any issues that arise from their use. In short, this principle answers the question “Who's Responsible When Things Go Wrong?”.
Examples of Accountability
- Financial Fraud Detection System: An AI system used by banks to detect fraudulent transactions might mistakenly flag legitimate transactions as suspicious. Accountability could lie with the developers for potential flaws in the AI model. The banks utilizing the system also hold some responsibility for verifying the accuracy of AI-flagged transactions and ensuring proper customer service processes.
- Self-Driving Car Accidents: An autonomous vehicle makes a faulty decision that results in an accident. Accountability could fall on several parties depending on the situation. The car manufacturer might be responsible if the accident stemmed from a flaw in the AI software. Data providers could be held accountable if faulty or biased data used to train the AI contributed to the accident. Additionally, regulators might need to review their oversight procedures for self-driving car technology.
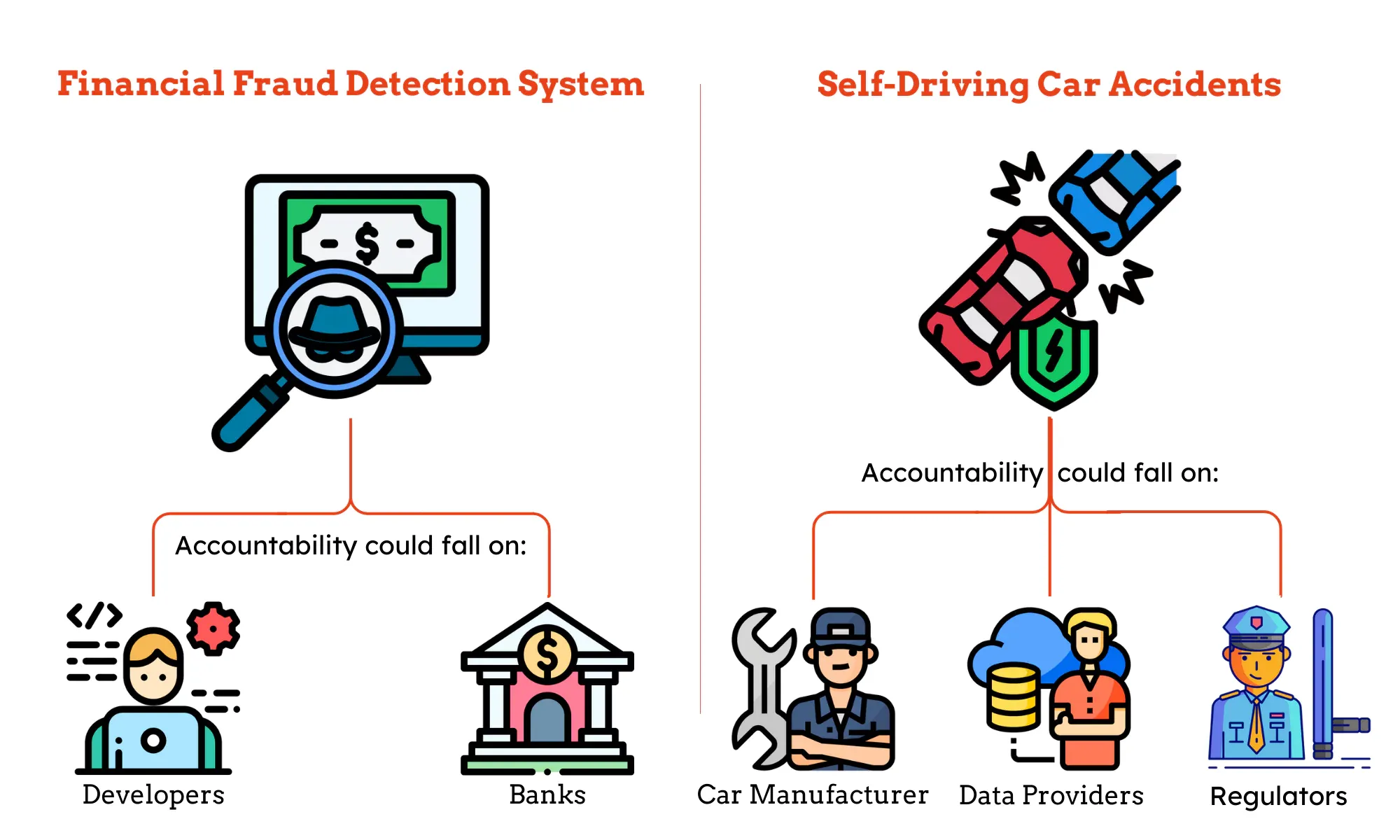
Strategies for Ensuring Accountability
- Clear Documentation: Maintain detailed documentation of AI systems, including their design, development processes, and decision-making criteria. This helps in understanding how the AI works and in identifying any potential issues.
- Regular Audits: Conduct regular audits of AI systems to check for compliance with ethical guidelines and regulations. These audits can help identify and address biases, errors, and other issues in AI systems.
- Impact Assessments: Perform impact assessments to evaluate the potential effects of AI systems on different stakeholders. These assessments can help in understanding and mitigating any negative impacts of AI systems.
- User Feedback Mechanisms: Implement mechanisms for users to provide feedback on AI systems. This feedback can be used to identify issues and improve the system over time.
- Transparent Reporting: Provide transparent reporting on the performance and impact of AI systems. This includes sharing information about any issues that have been identified and the steps taken to address them.
Principle 4: Privacy and Security
Privacy and security protects user data from unauthorized access, misuse, and breaches. It ensures that AI systems handle data responsibly, maintaining confidentiality and integrity throughout their lifecycle.
Security and Privacy Concerns
Security:
- Data Breaches: AI systems can be vulnerable to cyberattacks that steal or manipulate the data they rely on.
- Adversarial Attacks: Malicious actors might attempt to manipulate AI models with adversarial inputs, causing them to produce incorrect or harmful outputs.
- Algorithmic Hijacking: In some cases, attackers might exploit vulnerabilities in the AI algorithms themselves to gain control or manipulate their behavior.
Privacy:
- Data Collection: The vast amount of data collected for AI development raises concerns about user privacy and potential misuse of personal information.
- Data Inference: Even anonymized data can sometimes be used to infer sensitive information about individuals.
- Lack of Transparency: When users don't understand how their data is being used in AI systems, it can lead to privacy concerns and a lack of control over their information.
Strategies for Ensuring Privacy and Security
- Data Encryption: Encrypt data both in transit and at rest to protect it from unauthorized access. This ensures that even if data is intercepted or breached, it cannot be easily read or used.
- Access Controls: Implement strict access controls to limit who can access sensitive data. This includes using authentication methods such as passwords, biometrics, and multi-factor authentication.
- Data Anonymization: Anonymize data to remove personally identifiable information (PII) before using it for AI training and analysis. This helps protect user privacy while still allowing valuable insights to be gained from the data.
- Regular Security Audits: Conduct regular security audits and vulnerability assessments to identify and address potential security weaknesses in AI systems.
- Compliance with Regulations: Ensure that AI systems comply with relevant data protection regulations, such as GDPR (General Data Protection Regulation) and CCPA (California Consumer Privacy Act). This includes obtaining user consent for data collection and providing users with rights to access, correct, and delete their data.
Examples of Privacy and Security
- Healthcare Data Protection: An AI tool analyzing medical records should use data encryption and anonymization to ensure patient privacy. Regular audits should be conducted to comply with regulations like HIPAA (Health Insurance Portability and Accountability Act).
- Financial Transactions Security: Banks using AI for fraud detection must ensure that transaction data is securely encrypted and access is restricted. Compliance with financial regulations, such as the Payment Card Industry Data Security Standard (PCI DSS), is essential for maintaining security and trust.
- Smart Home Devices: AI-powered smart home devices, like voice assistants, must protect user data from unauthorized access. This can involve encrypting voice recordings and ensuring that only authorized users can access and control the devices.
>> Read more about AI:
- Benefits of Artificial Intelligence (AI) in Mobile App Development
- AI in Software Testing: How It Works, Benefits & Challenges
- Top 12 Best Free AI Chatbots for Businesses
- Top 5 Best Generative AI Tools
- Top 17 Best AI Apps For Individuals and Businesses
- Top 9 Best Deep Learning Frameworks for Developers
- Top 7 Machine Learning Solutions For Growing Your Business
Final Thoughts
The four key principles of Responsible AI – Fairness, Transparency, Accountability, and Security & Privacy – serve as a roadmap for developing and deploying AI in a way that benefits everyone. By adhering to these principles, we can partly ensure that AI systems are ethical, trustworthy, and fair.
While challenges remain, ongoing research and development in explainable AI, regulatory frameworks, and security measures hold immense promise for a future powered by more responsible and trustworthy AI.
- development