Ever dreamed of having a powerful AI assistant that can bring your creative ideas to life. Generative AI tools are here to transform the way you work. They leverage advanced machine learning to produce everything from compelling text to stunning images, and even self-writing code.
These cutting-edge tools harness the power of natural language processing (NLP) and other AI technologies to enhance productivity and creativity across various fields. Whether you're an experienced writer, a design expert, or a coding enthusiast, generative AI can significantly boost your workflow and open up a realm of creative possibilities.
Ready to dive in and explore these game-changing tools? Let's get started!
>> Read more:
- How Does No-Code AI Assist In App Development?
- No-Code And Low-Code Solutions In Logistics And Supply Chain
- Will AI Replace Software Engineers Altogether?
- What is the Responsibility of Developers using Generative AI?
What are Generative AI Tools?
Generative AI tools are advanced software applications that leverage artificial intelligence, particularly natural language processing (NLP) and machine learning, to create content that mimics human creativity and decision-making. These tools can generate text, images, audio, and even code, offering innovative solutions across various domains.
Here is how Generative AI tools work:
- Learning from Extensive Data: Generative AI models are trained on vast datasets that include text, code, and images. This extensive data training helps the models understand various subjects and contexts, forming the basis for their creative capabilities.
- Generating New Content: Once trained, these models can produce realistic and original outputs by combining learned patterns. They effectively use their "knowledge library" to create new and unique content, from text and images to code.
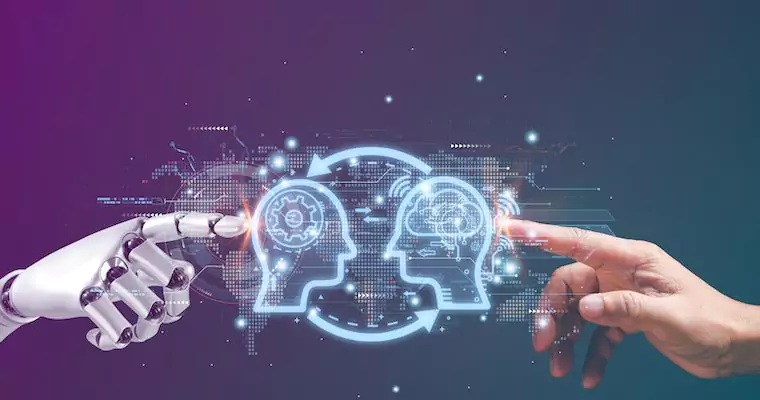
Different Types of Generative AI Tools
>> Read more: Top Mobile App Development Trends
Generative AI tools are diverse, each excelling in different creative domains. Here's a concise overview of the most popular types:
Text Generation
These tools can craft ad copy, product descriptions, fiction, scripts, blog post outlines, and social media captions. They brainstorm ideas and generate compelling text swiftly.
Image Generation
AI can turn your descriptions into stunning images, from fantastical landscapes to realistic portraits. These tools like an AI image generator, creating unique artistic styles and modifying existing images based on your input.
Video Generation
AI technology is also transforming the way we create videos. With an AI video generator, you can produce compelling video content from text prompts, images, or even voice input. These tools are perfect for marketers, educators, and creators looking to streamline video production while maintaining high quality and creativity.
Code Generation
Generative AI assists programmers by suggesting solutions, identifying bugs, and generating code snippets. It streamlines workflow and boosts productivity for both seasoned developers and beginners.
>> Read more: What is the Responsibility of Developers using Generative AI?
Music Generation
AI-powered tools compose music based on your mood or genre preferences, producing everything from background scores to complex orchestral arrangements.
Other Creative Applications
- 3D Modeling: Generate detailed 3D models for architectural designs or video game characters.
- Game Development: Create storylines and in-game environments with AI assistance.
- Data Augmentation: Generate synthetic data to enhance machine learning model training.
Generative AI tools are continuously evolving, offering innovative applications across various fields. This brief overview showcases the immense potential of these tools, and as technology advances, even more exciting possibilities will emerge.
Industries Which Are Currently Applying Generative AI Tools
Industries are employing generative AI tools in many ways that will grow as the technology and our understanding of it advance. Examples of current generative AI applications in many fields:
Entertainment and Media: Generative AI tools automate content generation for writers, marketers, and content creators, optimizing processes and saving 40% of content creation time. They may concentrate on strategic tasks and innovation with this efficiency boost.
Education: AI can develop course materials, lesson plans, online learning systems, and chatbots for one-on-one teaching.
Meteorology and Climate: AI can predict weather, simulate natural disasters, and model climate scenarios.
Art and Design: Artists and designers are pushing creativity by using generative AI tools to create new visual art, illustrations, and designs. A study indicated that using generative AI tools in design increased the quantity of creative and eye-catching design concepts by 75%.
Software Development: Generative AI is changing code writing. It helps developers generate code snippets, improve software testing by finding 30% more flaws, and find optimal coding solutions. Higher code quality and faster development cycles arise from these characteristics.
Translation: Real-time generative AI tools translate text and speech, enabling multilingual communication. Global communication and comprehension are enhanced by these products' 95% translation accuracy.
Healthcare: Generative AI analyzes medical imagery, diagnoses diseases, and predicts patient outcomes. Radiologists employing generative AI tools for image processing detected tiny anomalies 20% more accurately, leading to faster and more accurate diagnoses.
Gaming: Game developers use generative AI tools to create immersive virtual worlds, produce in-game content, and adapt gameplay to player activity. Dynamic adaptation boosts player involvement and pleasure by 50%, improving the gaming experience.
Finance: Financial organizations use generative AI tools to study market trends, anticipate stock movements with 85% accuracy, and optimize trading methods. This technology-driven approach has increased trading profitability by 25% and improved investment selections.
>> Read more:
- An In-Depth Guide to Web Application Security for Businesses
- 5 Best Practices for Enhancing CI/CD Pipeline Security
Top 5+ Best Generative AI Tools in 2024
GPT-4
After the success and broad acceptance of GPT-3 and GPT-3.5, OpenAI created GPT-4, its latest Large Language Model (LLM). GPT-4 is said to be more innovative, accurate, safe, and stable than prior versions.
GPT-4 improves AI language models' text production across domains. Writing, marketing, and education benefit from its content generating skills. Data analysis is improved by its versatility in natural language processing.
Key Features:
-
Has 100 trillion parameters.
-
Large multimodal model.
-
Accepts both image and text inputs.
-
Emits text-only outputs.
-
Engine behind ChatGPT Plus.
-
Available via ChatGPT and API waitlist.
-
Multilingual capability.
-
Produce more coherent and nuanced responses.
Pros:
-
This latest GPT release is more stable than prior ones.
-
GPT-4 did well on standardized exams, such as the bar exam and numerous AP tests.
-
GPT-4 features more safety checks and training than earlier versions, including pre-training data filtering, expert reviews, model safety improvements, and frequent monitoring.
Cons:
-
Public image inputs are unavailable.
-
Questions remain concerning the technology's stability and safety.
-
Still sensitive to training data biases.
-
GPT-4 has improved but still has bias in several outputs.
Pricing:
-
Free Version.
-
Prompt: $0.03/1,000 tokens.
-
Completion: $0.06/1,000 tokens.
-
Paid membership: $20/month.
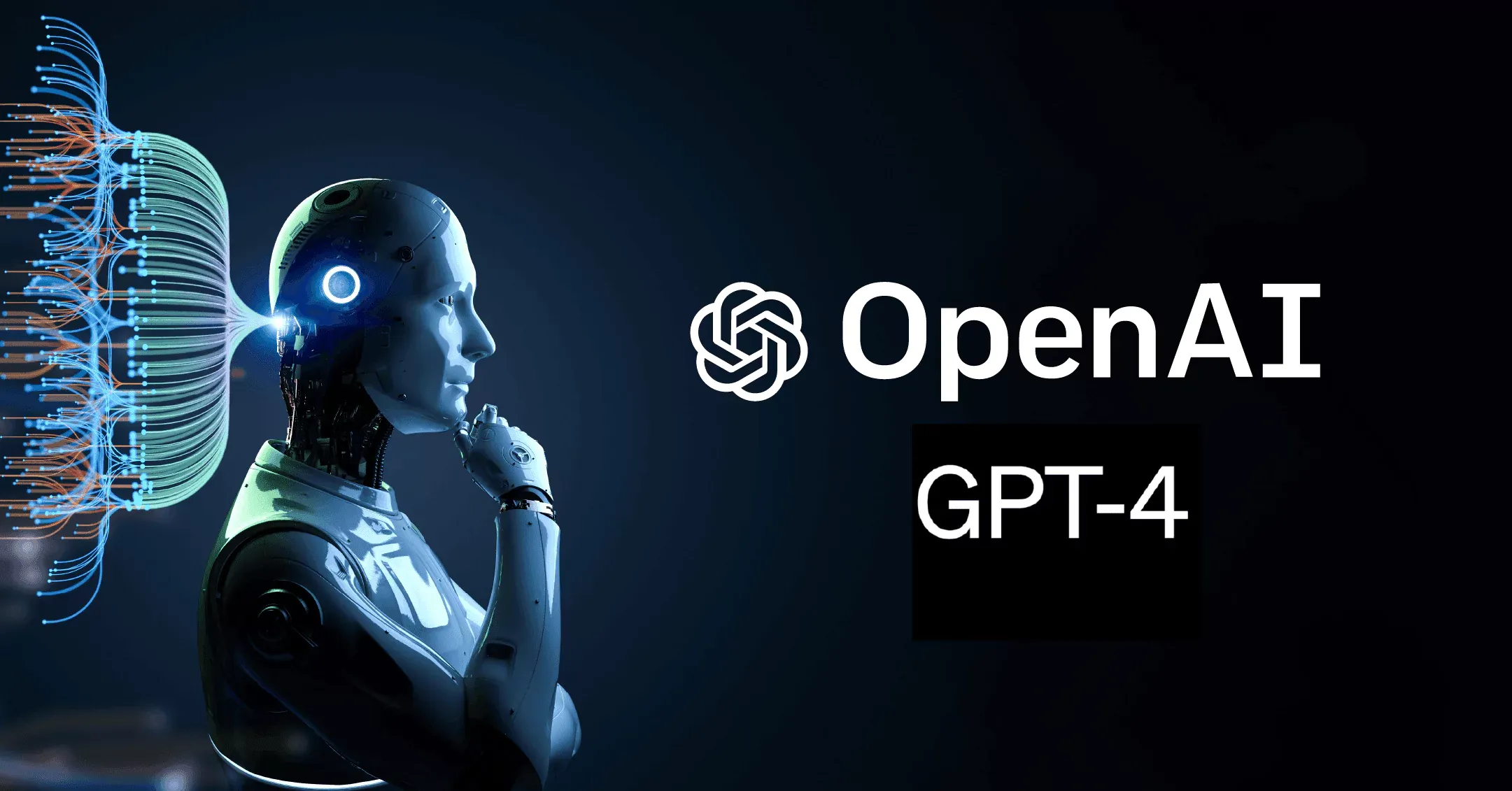
Bard
Bard, Google's reaction to ChatGPT, is a transformer-based chatbot and content generator based on LaMDA. Since the tool is still technically an experiment, Google is only making it available to a small subset of people in the US and UK for now.
The tool has fewer functionality than ChatGPT, but it's expanding. Coding and development necessities such as debugging, generating, and explaining code are all things that Bard was enhanced to handle in late April 2023.
The AI Bard chatbot from Google combines the world's information with massive language models' power, intellect, and creativity. It uses web information to provide fresh, high-quality responses, is capable of following instructions and completing requests thoughtfully, answering questions, and generating poems, code, scripts, musical pieces, email, and letters.
Key Features:
-
Based on transformer-based Language Model for Dialogue Applications (LaMDA).
-
Response rating by users.
-
Accessed via personal Google accounts.
-
Waitlisted for limited US and UK users.
-
Supports programming and software development.
Pros:
-
A result of Google's ethical and transparent AI development.
-
Thousands of testers trained and gave Bard comments before its launch.
-
Bard uses the internet, therefore its responses are less likely to be obsolete than ChatGPT's.
Cons:
-
ChatGPT has conversational history, while Bard does not.
-
Users with Google Workspace admin accounts cannot use this feature.
-
Bard is solely in English, while ChatGPT is multilingual.
Pricing: Free (limited users).
Claude
The $5 billion AI business Anthropic offers Claude, one of its latest chatbot aides and content creators. The program is similar to ChatGPT but focuses on safety and a customizable, conversational tone. Early users lauded Claude's comedy, content creation, and communication style analysis.
Quora, DuckDuckGo, Robin AI, and Juni Learning were early Claude testers. Claude helped build Notion AI, the generative AI assistant integrated to Notion project management.
Key Features:
-
Based on Constitutional AI.
-
Available in Slack.
-
The product has two versions: Claude Instant and Claude Classic.
-
Developers can use backend API.
-
Support for many popular spoken languages and programming languages.
-
Processing large amounts of text data well.
-
Natural, fluid discourse.
-
Automates difficult workflows.
-
User feedback is seamlessly adapted for continual improvement.
Pros:
-
Possibly bigger context window than OpenAI models.
-
Claude has red-team prompts to prevent hazardous content.
-
Sometimes Claude seems self-aware and gives users detailed explanations when it cannot answer a question.
Cons:
-
If the user contextualizes their request as fiction, harmful requests can pass.
-
No free version of Claude exists.
-
Internet access is unavailable to Claude.
-
Its complexity makes it difficult.
Pricing:
Claude Instant:
-
Prompt: $1.63/ million tokens.
-
Completion: $5.51/ million tokens.
Claude-V1:
-
Prompt: $11.02/ million tokens.
-
Completion: $32.68/ million tokens.
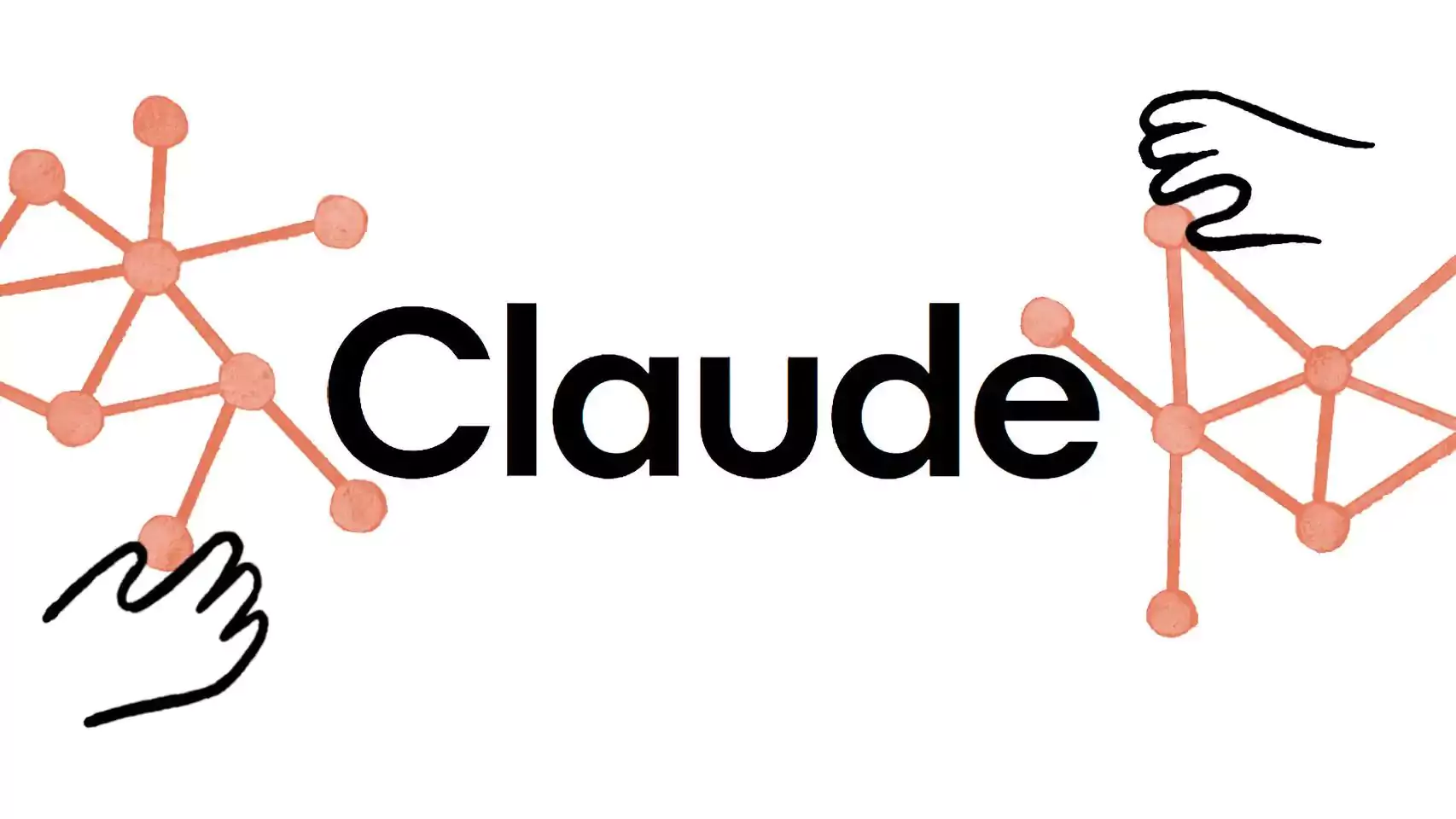
Synthesia
The AI video creation platform Synthesia lets users make videos using programmed prompts. The application then uses its AI avatars, voices, and video templates to generate a lifelike video. This tool requires no video editing expertise or equipment.
Product marketing, training, and how-to videos for internal and external users are most often created with Synthesia. For beginners, Synthesia offers example videos, a support center, and Synthesia Academy tutorials.
Key Features:
- The AI-powered platform rapidly translates text to videos.
- There are over 125 AI avatars.
- There are 120+ languages and accents.
- Users can construct AI dynamic avatars and scenes.
- AI-powered voice synthesis for smooth audio.
- Visuals, text, and voice can be customized.
- Users can choose pre-made video templates.
- Quickly create several videos simultaneously.
- Intercom, Mindstamp, PowerPoint, WordPress, Vimeo, and HubSpot Knowledge Base integrate.
Pros:
- Customizes videos to individual preferences by extensive options offered.
- Offers one free AI video to potential buyers.
- Videos can be made in 120+ languages.
- Users find the tool simple and accessible.
Cons:
- Authoring environment bugs can occur.
- Without the premium plan, audio is a little off.
- Some users have reported support issues.
- Videos can lack art without human contribution.
Pricing:
Personal:
- ₹1499.92/month, ₹17,999.00 billed annually, or pay monthly.
- $30 per month, billed monthly.
Enterprise: price based on the number of seats.
DALL-E 2
As a research project, OpenAI developed DALL-E in January 2021, which can create graphics using natural language. It introduced DALL-E 2, which combined concepts, attributes, and styles, a year later.
DALL-E 2 is OpenAI's latest picture and art-generating AI tool. Users can prompt DALL-E 2 to make a new image or edit an existing image.
DALL-E 2 generates more lifelike graphics that match user requests than DALL-E. Additionally, DALL-E 2 appears to have gotten greater training on how to reject unsuitable inputs and prevent problematic outputs than its predecessor.
Image synthesis is Dall-E2's forte. Translating words into appealing graphics allows artists and designers to experiment. Unique artwork and personalized images are notable triumphs. It bridges the text-image gap and spurs innovation despite occasional mistakes and limits in its learned notions.
Key Features:
- Phased learning deployment.
- Natural language inputs for producing visuals and art outputs.
- Multiple variations on the same image are possible.
- With the inpainting function, users can fix up old photos.
- The inpainting function is used to make requested changes or tweaks to an image.
- DALL-E was designed for programmers.
Pros:
- The resolution of DALL-E 2 is four times that of DALL-E.
- Developers can now add DALL-E to apps and products.
- Increases efficiency and generates varied images smoothly.
- Connects text and images.
- Inspires user creativity.
- Visual concepts may be prototyped quickly.
Cons:
- Creates incorrect images.
- Pre-trained image ideas limit.
- Possible misinterpretation of difficult text.
- Limited real-world accuracy, needing manual corrections.
Pricing: $15 USD/ 115 credits
>> Read more:
- Roadmap To Become A Machine Learning Engineer
- Cursor vs Copilot: Which is A Better AI-Powered Coding Tool?
Conclusion
Generative AI has opened up new possibilities, where machines are actively creating rather than just copying humans. The generative AI tools mentioned in this blog demonstrate technology's rapid advancement in code snippet generation, music composition, and visual content creation.
We must embrace generative AI's power while acknowledging its limitations and need for human monitoring and improvement. These advanced generative AI tools are helping industries achieve unparalleled efficiency, creativity, and innovation.
>>> Follow and Contact Relia Software for more information!
- Web application Development
- Designing an application
- development