Let's imagine a world where computers can make decisions that affect our lives, but we have no idea why. This is the reality with some Artificial Intelligence (AI) models. They can be powerful tools, but their lack of transparency can be unsettling.
Here’s where Explainable AI (XAI) comes in. It’s like having a window into the AI’s mind, letting us understand how it reaches its conclusions. Explainable AI (XAI) refers to methods and techniques used to make the outputs of AI systems understandable to humans. This transparency enables users to comprehend, trust, and effectively manage AI systems.
XAI is particularly important in sensitive domains, where understanding AI decisions can impact safety, fairness, and ethical considerations. Now, let's explore the key principles of XAI and the specific cases that benefit most from its implementation.
>> Read more:
- Dive Deep into The Four Key Principles of Responsible AI
- Benefits of Artificial Intelligence (AI) in Mobile App Development
4 Key Principles of Explainable AI
Explainable AI (XAI) relies on several core principles to ensure AI systems are transparent and understandable:
Transparency
The core principle states that an AI system should be able to provide reasons or evidence for its outputs. This explanation could take various form, depending on the complexity of the system and the intended audience.
Examples include highlighting the most important factors influencing a decision, providing counterfactual explanations (what would have happened if a single factor changed?), or offering visualizations of the model's internal workings.
Interpretability
Just providing an explanation isn't enough. It needs to be understandable to the intended user. This means tailoring the explanation to the user's level of expertise. Technical jargon might be appropriate for data scientists, but explanations for loan officers or patients should be clear and concise in everyday language.
Justifiability
This principle provides reasons and justifications for AI decisions, ensuring they are reasonable and can be explained logically to stakeholders. The explanation should accurately reflect the actual reasoning process of the AI model.
Reliability
No AI model is perfect, and XAI should acknowledge these limitations. The explanation should clarify the situations where the model is most confident and identify areas where its performance might be less reliable.
By adhering to these principles, XAI can ensure that explanations are not only provided but also informative, trustworthy, and tailored to the specific needs of the user.
>> Read more:
- Will AI Replace Software Engineers Altogether?
- What is the Responsibility of Developers using Generative AI?
6 Popular Cases Would Benefit from Explainable AI Principles
Explainable AI can shed light on how AI systems arrive at their conclusions. This transparency is particularly valuable in situations where understanding the "why" behind a decision is crucial. Here, we'll explore some key areas where XAI shines:
Healthcare
The first use case of Explainable AI is a healthcare scenario where an AI system assists doctors in diagnosing complex illnesses. This system wouldn't just provide a diagnosis; it would leverage XAI principles to empower both doctors and patients.
Example: A Healthcare AI System Used for Diagnosing Medical Conditions
Here's how it incorporates all the XAI principles:
- Transparency: The system provides clear documentation and visualizations of its algorithms, showing how it processes patient data to reach a diagnosis.
- Interpretability: It uses simple charts and explanations to show how symptoms and medical history lead to specific diagnoses, making it easy for doctors and patients to understand.
- Justifiability: The system gives logical reasons for each diagnosis, explaining which symptoms and factors contributed most to its decision.
- Reliability: It consistently produces accurate diagnoses backed by clinical trials and historical data, ensuring trust in its recommendations.
By combining these principles, the AI system helps doctors make informed decisions, improves patient trust, and enhances the overall quality of healthcare.
❗Caution: While XAI holds immense potential in healthcare, it's crucial to maintain a realistic perspective. XAI is a rapidly developing field, and its widespread adoption in healthcare is still in its early stages and challenging. Here are some key points to consider:
- Ongoing Research: Many XAI techniques are under active research and development. While promising, they might not be readily available or fully optimized for real-world healthcare applications yet.
- Data Quality: The effectiveness of any AI system, including those incorporating XAI principles, heavily relies on the quality of data used for training. Ensuring robust and unbiased datasets remains a challenge in healthcare.
- Regulation and Standards: Clear guidelines and regulations are needed to ensure the responsible implementation of XAI in healthcare. These standards are still being established.
In healthcare, where decisions can have profound consequences, it's crucial for providers to be cautious and continually validate AI systems. Uncritical reliance on XAI for decision-making can lead to irreversible errors.
>> Read more:
- A Detailed Breakdown For Healthcare Software Development
- Key Benefits of Healthcare Automation Systems
- Data Security in Healthcare Software: Why Is It Important?
- Guide to Medicine Delivery App Development For Pharmacy Businesses
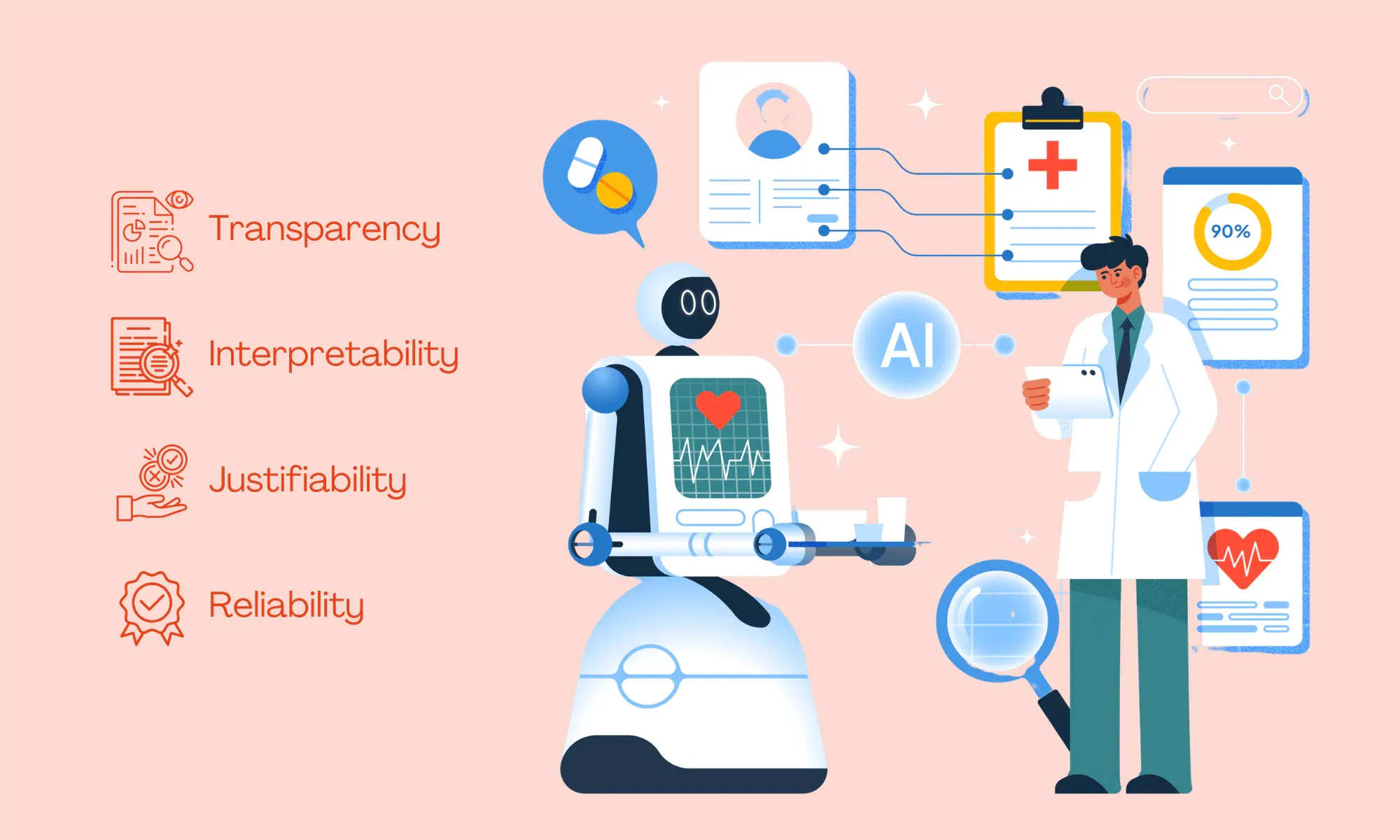
Finance
Explainable AI (XAI) principles are revolutionizing the finance industry, helping financial institutions make better decisions and improve their services. They can be used for tasks like loan approvals, fraud detection, and investment recommendations.
Example: A Loan Approval System
Traditionally, the bank might simply approve or deny your request, leaving you wondering about the rationale behind their decision. Here's how XAI principles can change that:
- Transparency: The bank uses a clear and accessible system that explains the factors considered by the AI model in your loan application. This involves a breakdown of how the model weighs your income, credit score, and employment history.
- Interpretability: The system goes beyond a simple "yes" or "no." It provides a detailed explanation of how your individual situation scored on each factor. For example, it might highlight a recent dip in your credit score as a key factor influencing the decision.
- Justifiability: The system offers clear justifications for the decision. If your loan is denied, the explanation details the specific reasons and suggests ways to improve your application for the future. This empowers you to take action and potentially increase your chances of loan approval next time.
- Reliability: The bank demonstrates the AI model's effectiveness through historical data, showing how accurately the model has predicted loan repayments in the past. This builds trust in the AI's capabilities and the overall loan approval process.
By incorporating XAI principles, the bank fosters a more transparent and fair loan approval process. This transparency builds trust and empowers you to make informed financial decisions.
❗Caution: While XAI has potential for fostering trust and fairness in financial decision-making, it's important to acknowledge some ongoing challenges.
Here are some key points to consider:
- Complexity of AI Models: Financial AI models can be highly intricate, making it difficult to explain their reasoning in a way that is easily understandable, especially for non-experts.
- Data Biases: If the data used to train the AI model is biased, the explanations generated by XAI might perpetuate those biases. Ensuring fair and unbiased data remains a crucial challenge.
- Security and Privacy Concerns: Explaining how an AI model works might inadvertently reveal sensitive information about its inner workings. Balancing transparency with security and privacy is an ongoing discussion.
>> Read more:
- Digital Transformation in Banking and Financial Services
- A Detailed Guide To FinTech App Development For Developers
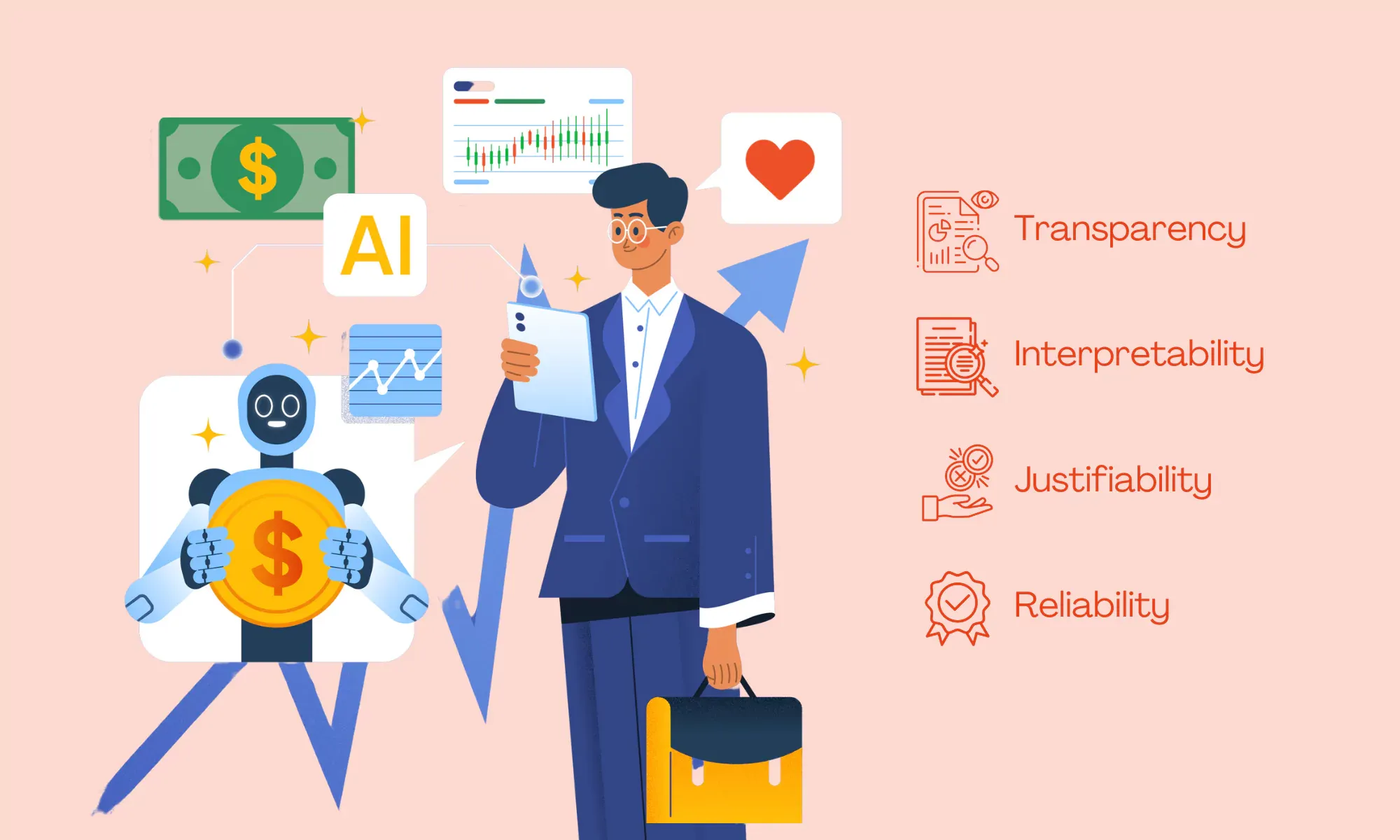
Legal Systems
The legal system thrives on transparency, fairness, and a clear understanding of the reasoning behind decisions. Explainable AI (XAI) principles can bring significant improvements to legal systems by ensuring fair and transparent decision-making processes. It helps legal professionals understand AI-driven decisions and provides clear justifications, building trust in AI applications in the legal field.
Example: A Sentencing Decision Support System
- Transparency: The AI system provides clear documentation and visualizations of the factors it considers in sentencing decisions. This might include the severity of the crime, the defendant’s criminal history, and other relevant factors.
- Interpretability: The system offers detailed explanations for each decision. For instance, it explains how the defendant's previous offenses and the nature of the current crime contributed to the recommended sentence.
- Justifiability: The system justifies its recommendations with clear reasons. If a defendant receives a harsher sentence, the explanation details why certain factors, such as repeated offenses or lack of remorse, led to this outcome. This helps judges understand and trust the AI’s suggestions.
- Reliability: The system's effectiveness is demonstrated through historical data, showing how well it aligns with actual sentencing patterns and outcomes. It might highlight its success rate in predicting recidivism or ensuring consistent sentencing for similar cases.
By incorporating XAI principles, the legal system can enhance the transparency and fairness of sentencing decisions. This approach builds trust among judges, lawyers, and the public, ensuring that AI contributes positively to the judicial process.
❗Caution:
- Bias and Fairness: AI systems can perpetuate existing biases present in the data they are trained on, leading to unfair or discriminatory legal decisions. Continuous monitoring and updating of AI models are necessary to mitigate this risk.
- Accountability: Determining responsibility for AI-driven decisions can be complex. Clear guidelines are needed to address who is accountable when AI recommendations lead to unjust outcomes.
- Ethical Considerations: Ensuring AI respects the rights and dignity of individuals is crucial. Ethical standards and guidelines must govern the use of AI in legal contexts.
- Transparency vs. Complexity: While XAI aims for transparency, the inherent complexity of some AI models can make full interpretability challenging. Legal professionals need to balance the need for understandable explanations with technical limitations.
- Evolving Legal Standards: The legal field is dynamic, and AI systems must be regularly updated to reflect current legal standards and practices to remain accurate and relevant.
- Public Trust and Acceptance: Building trust in AI systems within the legal community and the public is essential. Any misstep can lead to resistance and skepticism towards AI adoption in legal settings.
By addressing these challenges and exercising caution, the legal system can responsibly integrate XAI to improve decision-making processes while maintaining fairness and accountability.
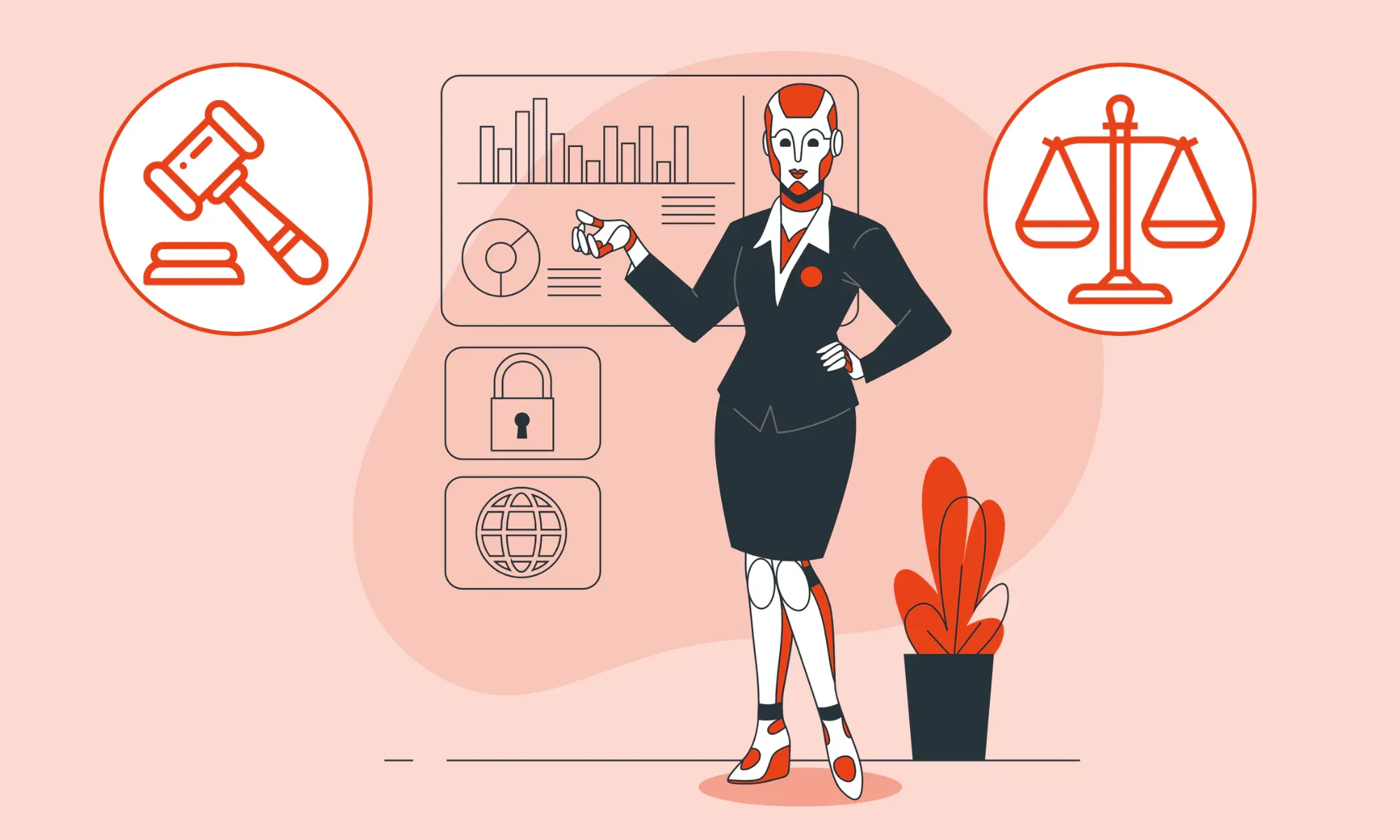
Autonomous Systems
Autonomous systems are machines that can sense their environment, make decisions, and act without direct human intervention. Explainable AI (XAI) is crucial in these autonomous systems, such as self-driving cars and drones, to ensure safety, reliability, and public trust. By providing clear explanations for AI-driven decisions, XAI enhances transparency and accountability in these high-stakes applications.
Example: A Self-Driving Car Navigating Through City Traffic
- Transparency: The AI system provides clear visualizations and documentation of how it processes sensor data to make driving decisions. This includes real-time displays showing the car's perception of its surroundings, including detected vehicles, pedestrians, and traffic signals.
- Interpretability: The system offers detailed explanations for its actions. For example, it can explain why it decided to brake suddenly by highlighting detected obstacles or changes in traffic signals.
- Justifiability: The car offers clear justifications for its maneuvers. If it needs to make an emergency stop, it should explain why (e.g., detected a pedestrian crossing) and potentially offer the driver the option to intervene.
- Reliability: The manufacturer demonstrates the AI model's effectiveness through rigorous testing and real-world data. This could involve showcasing the model's performance in various driving scenarios and its ability to handle unexpected situations. It might show a high success rate in avoiding accidents and following traffic regulations.
❗Caution:
- Real-World Complexity: The real world is messy and unpredictable. Explaining how an autonomous system like a self-driving car makes decisions in complex, ever-changing environments can be challenging. XAI needs to account for these complexities while remaining clear and concise.
- Sensor Limitations: Sensor limitations in autonomous systems can lead to inaccurate data, impacting the reliability of XAI explanations. Ensuring robust and high-fidelity sensor data is crucial for trustworthy explanations.
- Over-reliance: XAI explanations are valuable tools, but they shouldn't replace human oversight and safety protocols. Engineers and regulators still need to ensure the overall safety and reliability of autonomous systems through rigorous testing and fail-safe mechanisms.
- Ethical Dilemmas: Autonomous systems may face ethical decisions, such as prioritizing the safety of different individuals in unavoidable accident scenarios. XAI must address these dilemmas transparently, but clear ethical guidelines are essential.
- Public Perception: Gaining public trust is crucial for the adoption of autonomous systems. Any incidents involving AI-driven decisions can lead to public skepticism.
- Regulatory Compliance: Autonomous systems must comply with various regulations that may differ across regions. XAI systems need to be adaptable to meet these diverse requirements and ensure compliance.
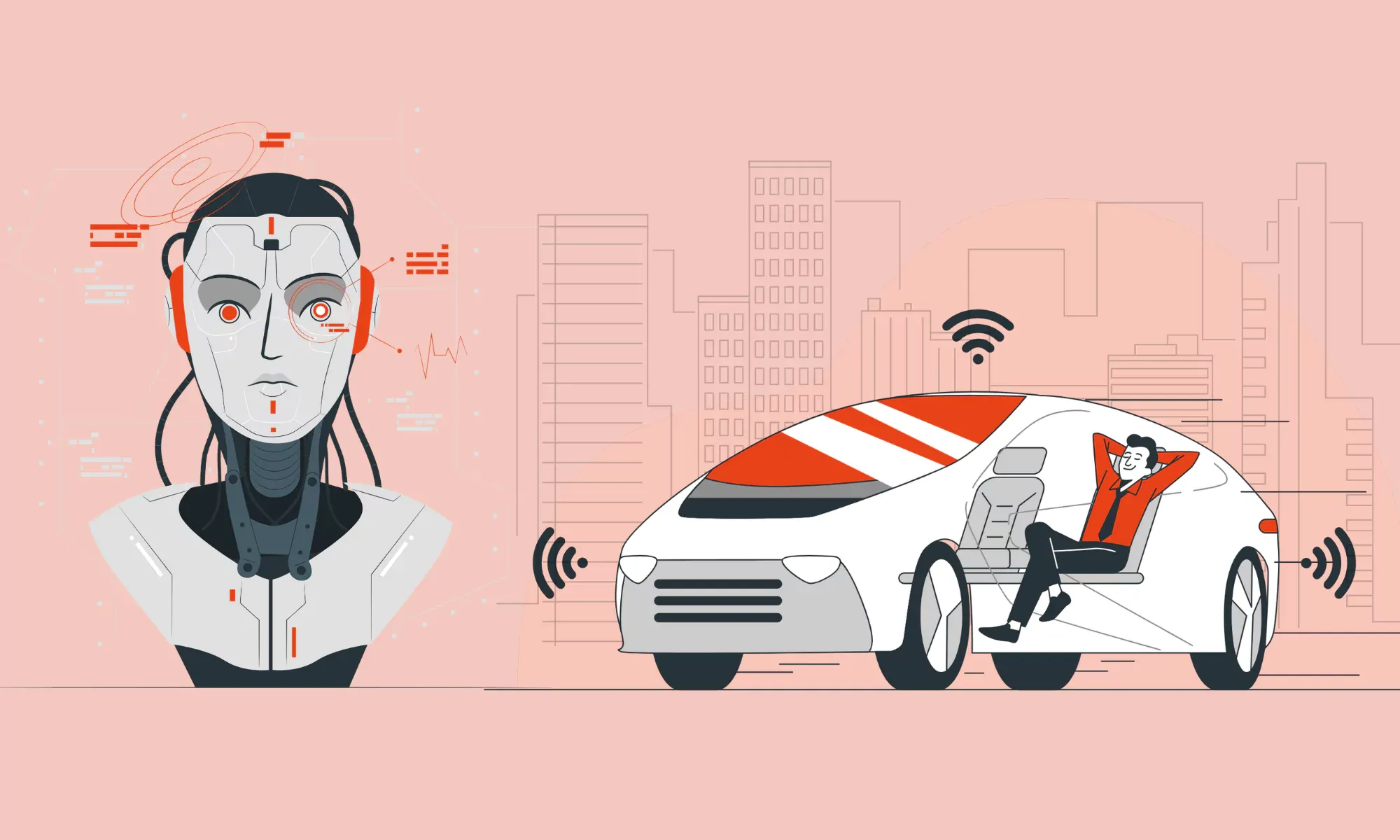
Customer Services
Explainable AI (XAI) principles enhance customer services by making automated processes transparent and understandable. This improves customer satisfaction and trust in AI-driven interactions.
Example: A Customer Support Chatbot
- Transparency: The chatbot clearly shows the steps it takes to resolve customer queries, such as the data it collects and how it processes requests. This might include visible prompts explaining why it asks specific questions.
- Interpretability: The chatbot provides detailed explanations for its responses. For instance, if it recommends a solution, it explains how it arrived at that recommendation based on the customer's input and previous similar cases.
- Justifiability: The chatbot offers logical reasons for its suggestions. If it proposes a troubleshooting step, it justifies this by explaining how this step has resolved similar issues in the past. This helps customers understand and trust the chatbot’s advice.
- Reliability: The chatbot's effectiveness is demonstrated through performance metrics and user feedback. The company shows that the chatbot successfully resolves a high percentage of queries, backed by user satisfaction scores and historical data.
❗Caution:
- Complexity of Interactions: Customer queries can be complex and varied. Ensuring that AI explanations are clear and relevant to diverse customer needs can be challenging.
- User Expectations: XAI explanations should not create the impression that AI can handle every customer service scenario perfectly. Customers may become frustrated if they encounter limitations in the AI's capabilities. It's important to emphasize that XAI is a tool to empower human agents and improve overall service, not a replacement for human interaction.
- Data Privacy: Providing personalized service often involves using customer data. It's crucial to ensure XAI explanations don't reveal sensitive information about past interactions or customer profiles. Customers have the right to privacy, and XAI solutions need to be developed with data security in mind.
>> You may consider:
- Top 12 Best Free AI Chatbots for Businesses
- Top 5 Best Generative AI Tools
- Top 17 Best AI Apps For Individuals and Businesses
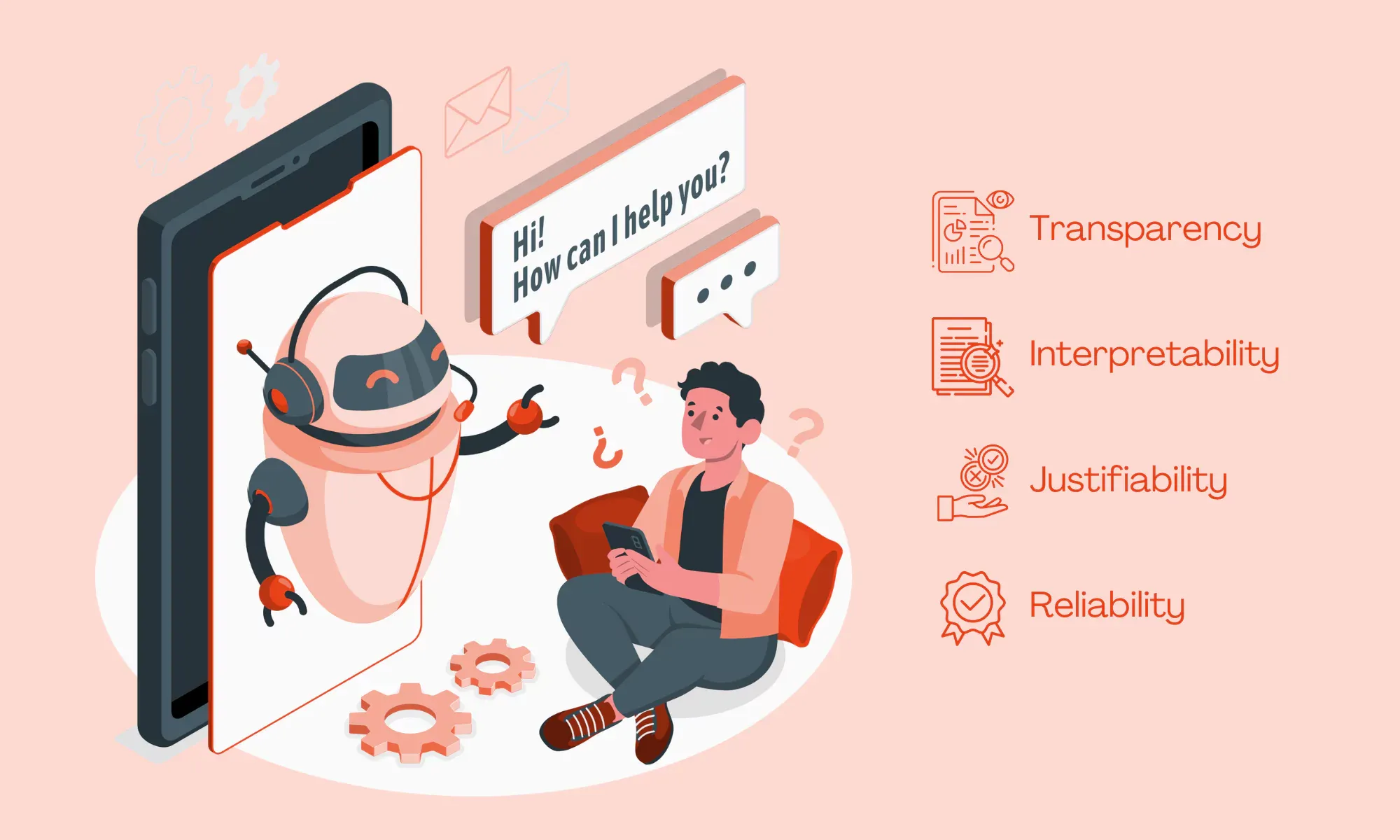
Software Development
Explainable AI (XAI) principles can significantly benefit software development by improving debugging, enhancing collaboration, and ensuring transparency in AI-driven development tools.
Example: An AI-Powered Code Review Tool
Suppose that you encounter a strange error message in your code. Traditionally, debugging might involve a lot of trial and error. Here's how XAI can change that:
- Transparency: The XAI system provides clear explanations for why the error occurred. This could involve highlighting the specific line of code that caused the issue and explaining how the program flow led to the error.
- Interpretability: The system goes beyond a simple error message. It offers insights into the program's state at the time of the error and the factors that contributed to it. This allows developers to understand not just the "what" but also the "why" behind the error.
- Justifiability: The system suggests potential solutions or code modifications to fix the bug. It explains the reasoning behind these suggestions, empowering developers to make informed choices about how to address the issue.
- Reliability: The XAI system builds trust by demonstrating its effectiveness through historical data. It might showcase how it has successfully helped identify and resolve similar bugs in the past.
By incorporating AI in software testing and debugging, developers gain valuable insights into the code's behavior, allowing them to fix bugs more quickly and prevent similar issues in the future.
❗Caution: While XAI holds promise in software development, there are important considerations:
- Complexity of Modern Software: Modern software has intricate dependencies, making it hard to explain bugs. XAI must balance comprehensive explanations without overwhelming developers.
- Interpretability and Developer Expertise: The utility of XAI depends on the developer's expertise. Complex AI concepts might be hard for less experienced developers to grasp, so explanations should match their skill level.
- Debugging Bias: AI models can be biased, highlighting popular errors more and potentially overlooking others. Ensuring unbiased training data is crucial.
- Security Concerns: XAI explanations might expose sensitive code details. Solutions must balance providing insights and safeguarding security.
- Evolving Technology: XAI systems must adapt to evolving software development practices. Regular updates are needed to maintain relevance.
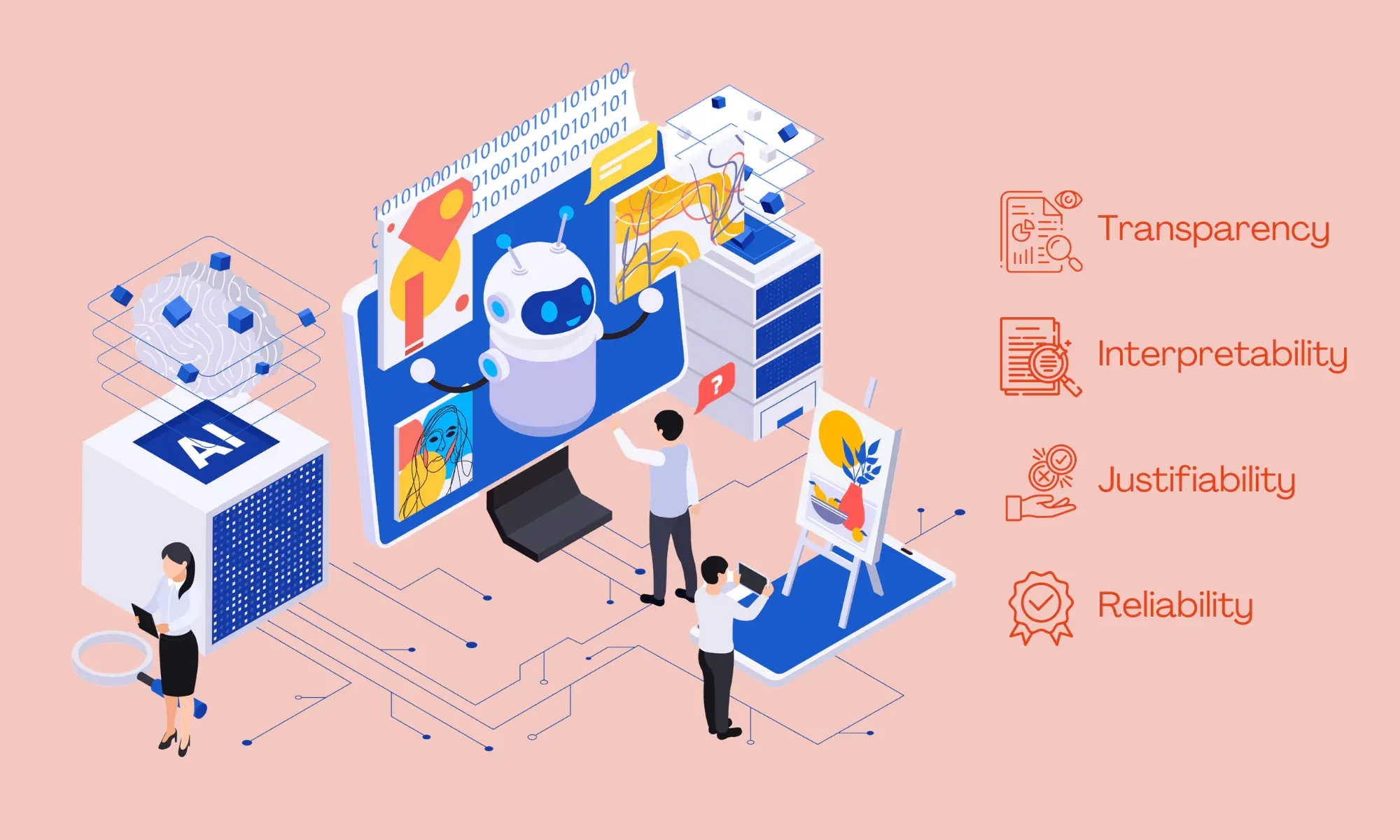
Final Thoughts
Explainable AI (XAI) is transforming various fields by applying 4 key principles, including transparency, interpretability, justifiability, and reliability. In healthcare, finance, legal systems, autonomous systems, customer services, and software development, XAI improves decision-making processes, fosters trust, and empowers users with clear explanations.
While XAI holds immense potential, it's crucial to address challenges such as bias, accountability, ethical considerations, and public trust. By continually refining and validating AI models, we can harness the full benefits of XAI, ensuring AI systems are transparent, fair, and reliable for all users.
- development