Thanks to innovative machine-learning techniques and AI, a new age in human-technology interaction is on the horizon. Computer programmers and software developers usually create artificial intelligence systems by using technologies such as machine learning, deep learning, neural networks, etc.
Of all those, Machine Learning is a rapidly developing area in the dynamic realm of technology. Machine learning uses powerful algorithms and statistical models to teach computers to learn and improve without being programmed.
This blog post will discuss promising machine learning technologies you should be aware of in 2024.
What are Machine Learning Solutions?
A machine learning (ML) solution is a comprehensive set of intellectual property, tools, and software enabling AI development across a wide range of platforms.
Fundamentally, machine learning enables various systems to learn from data and make judgments or produce other outputs based on inputs. Given the rate of innovation, machine learning solutions can quickly become fragmented or obsolete, and they may perform well for one particular device or environment but not for others, resulting in huge complexity.
A long-term machine learning solution allows AI development using a shared software architecture. It is also scalable, versatile, and power efficient in various cloud and edge computing settings.
4 Types of Machine Learning Solutions
A comprehensive machine learning solution can support all forms of AI & ML, such as supervised, unsupervised, semi-supervised, and reinforcement learning. Let's dive into 4 types of machine-learning solutions here!
Supervised Learning
This method entails training a model using labeled data that includes both input features and intended output labels. The model learns to link input features to their associated outputs, allowing it to make predictions on previously unknown data. (For example: spam filtering and image categorization).
Unsupervised Learning
Unlike supervised learning, unlabeled data is utilized. The model identifies patterns and structures in the data, enabling activities like data clustering, anomaly detection, and dimensionality reduction. (For example: customer segmentation and market research).
Reinforcement Learning
An agent interacts with its environment, gaining rewards for desired actions and penalties for undesirable ones. The agent learns by trial and error to maximize its rewards, making it suited for applications such as robotics control and gaming.
Semi-supervised Learning
It combines labeled and unlabeled data for training. The labeled data directs the learning process, whilst the unlabeled data enables the model to gain extra knowledge and improve performance.
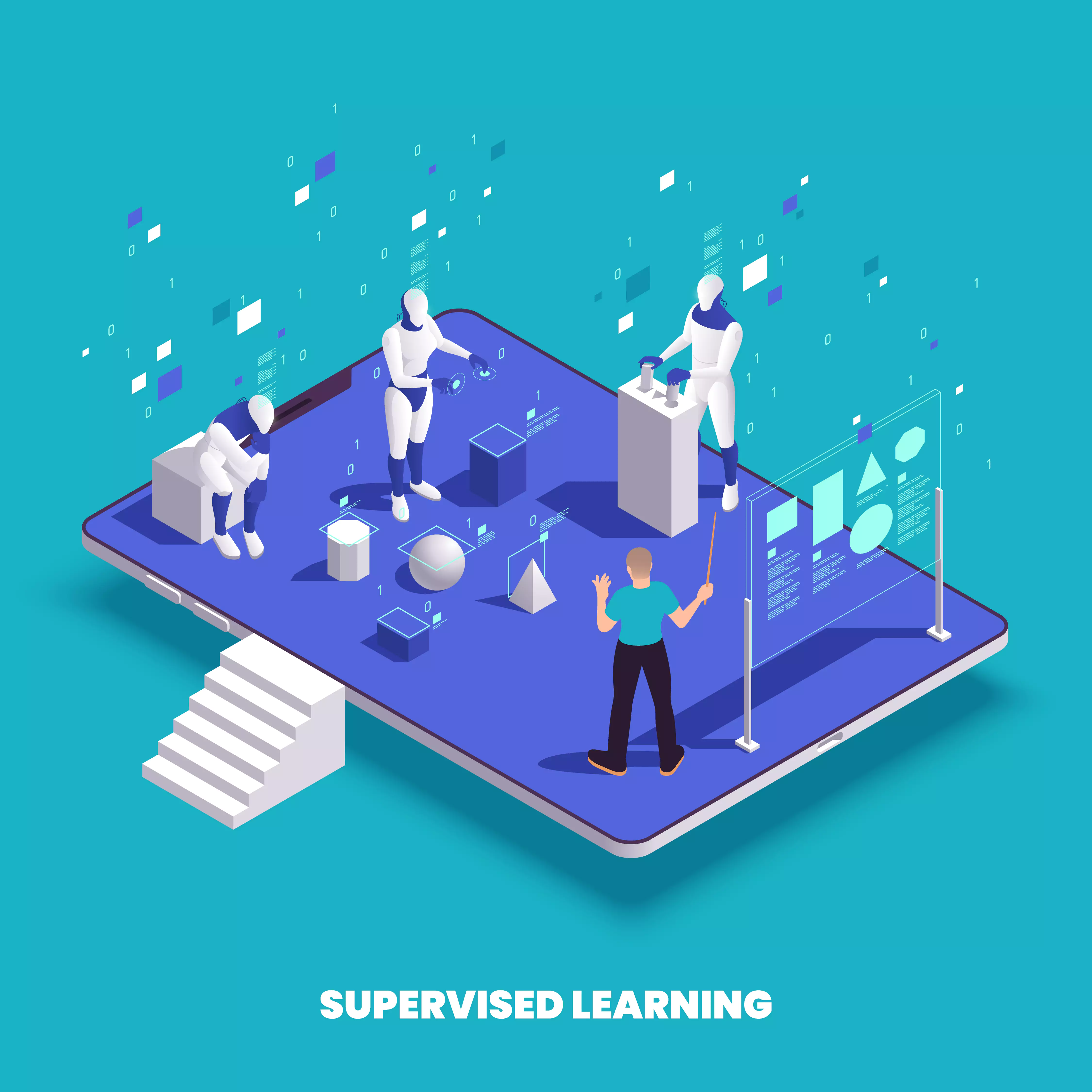
Benefits of Machine Learning Solutions
Machine learning solutions offer many advantages for businesses of all sizes. Here's a closer look at some of the key benefits:
Increased Efficiency & Automation
Machine learning is great at automating jobs that are done over and over again. This frees up valuable human resources to work on more important projects. Businesses can become much more efficient by automating tasks like data analysis, report creation, and even some decision-making. Machine learning can also automate chores that are currently done by hand and take a lot of time.
Improved Decision-Making Accuracy
Machine learning solutions can handle enormous volumes of data in real time. As a result, businesses can make better decisions with this data-driven method, which leads to more accuracy and efficiency.
Machine learning can be used to figure out why customers might leave, make marketing efforts more effective, and spot possible risks in financial transactions, among other things.
Enhanced Customer Experience
Machine learning solutions make the experience of the customer more unique by adapting exchanges and suggestions based on the person's past actions and preferences. This can be done with AI chatbots that offer personalized help, targeted marketing efforts, and product suggestions. Businesses can build better customer relationships and loyalty by giving customers a more relevant and interesting experience.
Furthermore, machine learning solutions can continually learn and adapt, enhancing their performance over time. This adaptability allows organizations to stay ahead of the competition by quickly responding to market trends and client preferences.
Reduced Costs
By automating chores, improving processes, and reducing mistakes, machine learning solutions can cut operational costs by a large amount. Machine learning can also help businesses make more money by helping them make better decisions and giving each customer a more personalized user experience. Machine learning gives companies a great return on their investment by cutting costs and bringing in more money.
Top 7 Machine Learning Solutions for Businesses
Cutting Edge Model Availability
As machine learning becomes more popular, free access to models is also growing. Parallel to machine learning development, huge companies are strengthening model performance standards. Their extensive datasets allow dedicated machine-learning solutions to train models.
Only a few companies have the funds or research technology to construct such models. Transfer learning allows them to build on or repurpose highly trained models to create high-performance models. Even huge companies have recognized the value of outside contributions to model development.
Students exploring machine learning solutions can use open-access or public models. Additionally, hobbyists and other groups can use these base models. Successful experiments may help these models and boost their careers.
Hyper-Automation
Hyper-automation suggests automating practically anything in a firm. It has been gaining popularity worldwide for some time, but last year's pandemic heightened its importance. Intelligent and digital process automation has increased.
Key segments of machine learning and artificial intelligence (AI) drive hyper-automation. Automated business operations must adapt to changing conditions and respond to sudden events.
Superior Supporting Tools for ML
Today, creating a working machine-learning model with decent predictions is not enough. Before opting to produce a model, machine learning solutions need model interpretability to comprehend predictions. This is critical for enterprises where predictions are evaluated for social justice, ethics, and fairness.
A key tool for model development is model cards, which are design documents that officially specify all model characteristics. These details are included:
- A detailed summary of the model's purpose.
- Author logistics connect to license, date, documents, etc.
- Neural network layer, input, and output specifications.
- Summary of its shortcomings, including ethical, privacy, speed, and accuracy difficulties.
- A performance metrics comparison between expected and actual accuracy.
Another important technique is visualization. Visualizing a model during design, training, and audit is invaluable. Team members utilize model cards to compare model performance to card specifications regularly.
Business Forecasting and Analysis
ML solutions corporate forecasting and decision-making. Machine learning engineers collect and analyze data over a given time to make wise conclusions. Machine learning can make 95% accurate conjectures after training with varied data sources.
We expect organizations to merge recurrent neural networks for accurate forecasts. Machine learning helps identify hidden patterns that were missed. Its best use is in insurance corporations to detect costly fraud. ML may help find hidden patterns and predict accurately.
Machine learning and Internet of Things (IoT)
Transforma Insights, an economic research firm, says that the IoT market will grow quickly and add 24.1 billion devices by 2030. This will bring in $1.5 trillion for the world economy.
The Internet of Things and machine learning are both used in different ways. Machine learning, artificial intelligence, and deep learning are used in the production of IoT products to make them smarter and safer. For the same reason, networks of IoT sensors and gadgets give machine learning and AI huge amounts of data they need to work well.
Machine Learning at the Edge
Business and consumer goods like the Google Mini leverage cloud-backed machine learning. Picture tiny gadgets that can connect to the internet and transfer data to the cloud for analysis in cloud-backed machine learning. In many cases, as when banks need to uncover fraud, latency isn't an issue. However, edge devices are obtaining the computational power they need to meddle.
Coral by Google is edge technology. It has an integrated TPU and solves many IoT use cases (speech and picture analysis). With the technology in a compact form factor, the inference is doable without the internet or cloud back end. By storing data on the device, machine learning at the edge provides application security.
Explainable AI (XAI)
As machine learning models get more complicated, it's important to understand how they make decisions. Explainable Artificial Intelligence (XAI) tries to give reasons that are easy to understand for the predictions that machine learning models make. It makes people more likely to trust the technology and lets everyone know why and how a choice was made.
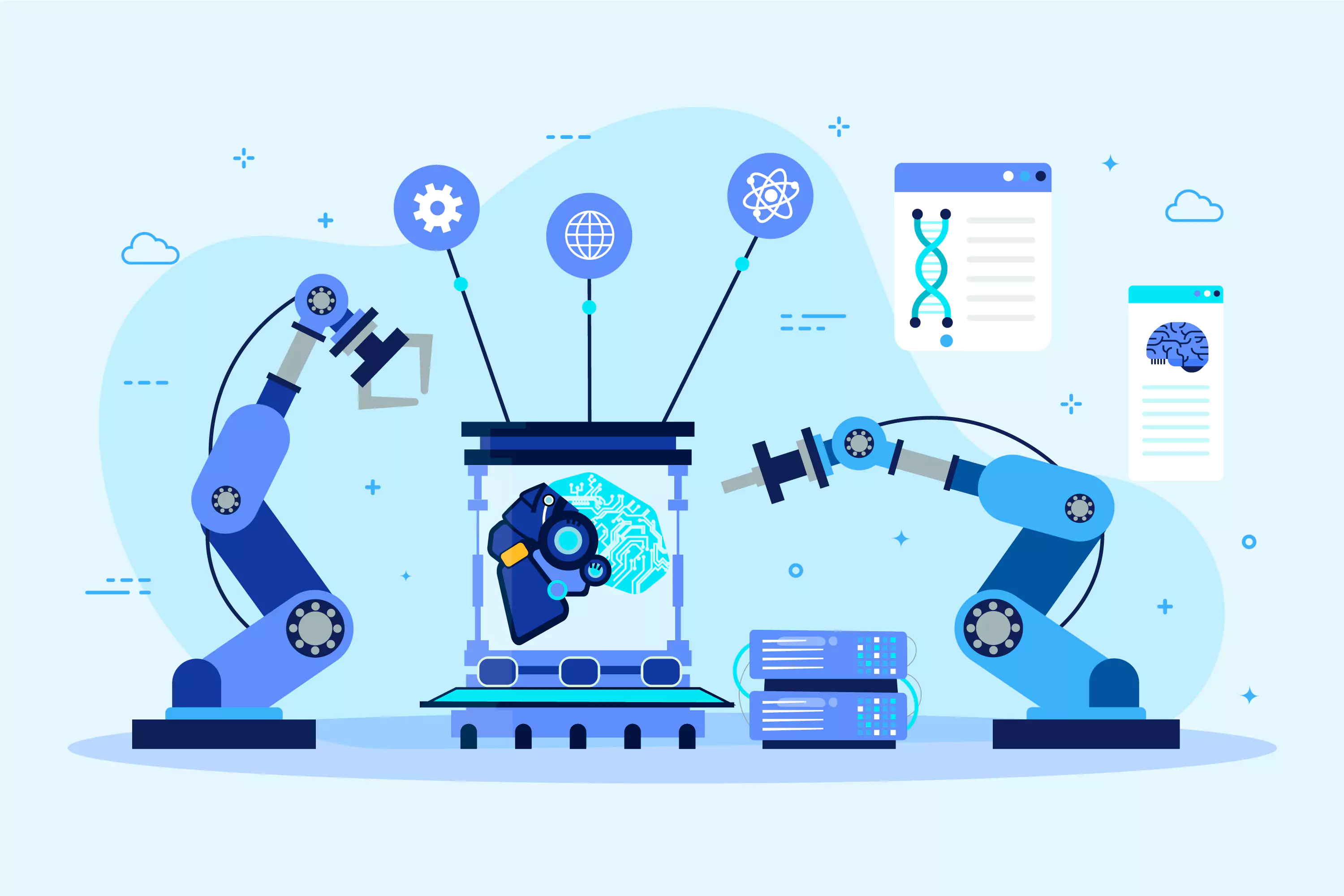
>> Read more:
- Top 9 Machine Learning Platforms for Developers
- Top 9 Best Deep Learning Frameworks for All Levels Developers
- ChatGPT, GitHub Copilot, and Tabnine: Top AI-Driven Tools
- Top 10 Best AI Development Tools for Software Developers
- What is Generative AI? 5 Best Generative AI Tools
How to Choose the Right Machine Learning Solutions?
Selecting the right machine learning solution is crucial for maximizing its benefits for your business. Here are some key factors to consider:
Identify Your Business Needs
The first step is to precisely outline the exact challenges or goals you wish to solve using machine learning. What aspects of your organization would benefit the most from automation, better decision-making, or increased personalization? Understanding your requirements will help you select a machine-learning solution that is well-suited to your business objectives.
Evaluate Data Availability & Quality
Machine learning algorithms thrive on data. As a result, it's critical to examine your current data resources. Do you have enough data volume and variety relevant to the problem you're trying to solve? Furthermore, ensure that the data is of good quality, accurate, complete, and error-free. Poor data quality can degrade the performance of any machine-learning solution.
Consider Scalability & Integration
Your machine-learning solutions should be able to scale as your business grows and the volume of data increases. Choose a solution that can accommodate greater data sets and changing requirements. Consider how the solution will work with your current IT infrastructure and other business processes. Seamless integration assures smooth operation and maximizes the return on your machine learning investment.
Choose a Reliable Vendor/Partner
The selection of a respected vendor or partner with machine learning experience is critical for effective implementation. Look for a vendor who provides a complete solution, which includes data preparation, model training, deployment, and continuing support. Choose a partner with a proven track record and a thorough understanding of your sector and its issues.
>> Read more: Why Should Python Be Used For Machine Learning?
Conclusion
Machine learning solutions transform industries and technology use. These machine learning solutions above will increase adoption, privacy and transparency, real-time decision-making, and domain possibilities. Acknowledge yourself with machine learning solutions to help your business grow and boost sales!
Relia Software can bring AI and machine learning solutions to your business with our dedicated developers and quality products. Let Relia help to boost your business productivity. Contact us!
>>> Follow and Contact Relia Software for more information!
- development